Flow cytometry#
You’ll learn how to manage a growing number of flow cytometry data batches as a single queryable dataset.
Specifically, you will
read a single
.fcs
file as anAnnData
and seed a versioned dataset with it (, current page)
append a new data batch (a new
.fcs
file) to create a new version of the dataset ()
Setup#
!lamin init --storage ./test-facs --schema bionty
Show code cell output
✅ saved: User(id='DzTjkKse', handle='testuser1', email='testuser1@lamin.ai', name='Test User1', updated_at=2023-10-10 15:45:12)
✅ saved: Storage(id='QK7Rd19J', root='/home/runner/work/lamin-usecases/lamin-usecases/docs/test-facs', type='local', updated_at=2023-10-10 15:45:12, created_by_id='DzTjkKse')
💡 loaded instance: testuser1/test-facs
💡 did not register local instance on hub (if you want, call `lamin register`)
import lamindb as ln
import lnschema_bionty as lb
import readfcs
lb.settings.species = "human"
💡 loaded instance: testuser1/test-facs (lamindb 0.55.2)
ln.track()
💡 notebook imports: lamindb==0.55.2 lnschema_bionty==0.31.2 pytometry==0.1.4 readfcs==1.1.7 scanpy==1.9.5
💡 Transform(id='OWuTtS4SAponz8', name='Flow cytometry', short_name='facs', version='0', type=notebook, updated_at=2023-10-10 15:45:17, created_by_id='DzTjkKse')
💡 Run(id='R7aH6AfJFQF8f46JiC2H', run_at=2023-10-10 15:45:17, transform_id='OWuTtS4SAponz8', created_by_id='DzTjkKse')
Ingest a first file#
Access
#
We start with a flow cytometry file from Alpert et al., Nat. Med. (2019).
Calling the following function downloads the file and pre-populates a few relevant registries:
ln.dev.datasets.file_fcs_alpert19(populate_registries=True)
PosixPath('Alpert19.fcs')
We use readfcs to read the raw fcs file into memory and create an AnnData
object:
adata = readfcs.read("Alpert19.fcs")
adata
AnnData object with n_obs × n_vars = 166537 × 40
var: 'n', 'channel', 'marker', '$PnB', '$PnE', '$PnR'
uns: 'meta'
It has the following features:
adata.var.head(10)
n | channel | marker | $PnB | $PnE | $PnR | |
---|---|---|---|---|---|---|
Time | 1 | Time | 32 | 0,0 | 2097152 | |
Cell_length | 2 | Cell_length | 32 | 0,0 | 128 | |
CD57 | 3 | (In113)Dd | CD57 | 32 | 0,0 | 8192 |
Dead | 4 | (In115)Dd | Dead | 32 | 0,0 | 4096 |
(Ba138)Dd | 5 | (Ba138)Dd | 32 | 0,0 | 4096 | |
Bead | 6 | (Ce140)Dd | Bead | 32 | 0,0 | 16384 |
CD19 | 7 | (Nd142)Dd | CD19 | 32 | 0,0 | 4096 |
CD4 | 8 | (Nd143)Dd | CD4 | 32 | 0,0 | 4096 |
CD8 | 9 | (Nd144)Dd | CD8 | 32 | 0,0 | 4096 |
IgD | 10 | (Nd146)Dd | IgD | 32 | 0,0 | 8192 |
Transform: normalize
#
In this use case, we’d like to ingest & store curated data, and hence, we split signal and normalize using the pytometry package.
import pytometry as pm
First, we’ll split the signal from heigh and area metadata:
pm.pp.split_signal(adata, var_key="channel", data_type="cytof")
'area' is not in adata.var['signal_type']. Return all.
adata
AnnData object with n_obs × n_vars = 166537 × 40
var: 'n', 'channel', 'marker', '$PnB', '$PnE', '$PnR', 'signal_type'
uns: 'meta'
Normalize the dataset:
pm.tl.normalize_arcsinh(adata, cofactor=150)
Note
If the dataset was a flow dataset, you’ll also have to compensate the data, if possible. The metadata should contain a compensation matrix, which could then be run by the pytometry compensation function. In the case here, its a cyTOF dataset, which doesn’t (really) require compensation.
Validate: cell markers
#
First, we validate features in .var
using CellMarker
:
validated = lb.CellMarker.validate(adata.var.index)
❗ 13 terms (32.50%) are not validated for name: Time, Cell_length, Dead, (Ba138)Dd, Bead, CD19, CD4, IgD, CD11b, CD14, CCR6, CCR7, PD-1
We see that many features aren’t validated because they’re not standardized.
Hence, let’s standardize feature names & validate again:
adata.var.index = lb.CellMarker.standardize(adata.var.index)
validated = lb.CellMarker.validate(adata.var.index)
❗ 5 terms (12.50%) are not validated for name: Time, Cell_length, Dead, (Ba138)Dd, Bead
The remaining non-validated features don’t appear to be cell markers but rather metadata features.
Let’s move them into adata.obs
:
adata.obs = adata[:, ~validated].to_df()
adata = adata[:, validated].copy()
Now we have a clean panel of 35 validated cell markers:
validated = lb.CellMarker.validate(adata.var.index)
assert all(validated) # all markers are validated
Register: metadata
#
Next, let’s register the metadata features we moved to .obs
.
For this, we create one feature record for each column in the .obs
dataframe:
features = ln.Feature.from_df(adata.obs)
ln.save(features)
We use the Experimental Factor Ontology through Bionty to create a “FACS” label:
lb.ExperimentalFactor.bionty().search("FACS").head(2) # search the public ontology
ontology_id | definition | synonyms | parents | molecule | instrument | measurement | __ratio__ | |
---|---|---|---|---|---|---|---|---|
name | ||||||||
fluorescence-activated cell sorting | EFO:0009108 | A Flow Cytometry Assay That Provides A Method ... | FACS|FAC sorting | [] | None | None | None | 100.0 |
FACS-seq | EFO:0008735 | Fluorescence-Activated Cell Sorting And Deep S... | None | [EFO:0001457] | RNA assay | None | None | 90.0 |
We found one for “FACS”, let’s save it to our in-house registry:
# import the FACS record from the public ontology and save it to the registry
facs = lb.ExperimentalFactor.from_bionty(ontology_id="EFO:0009108")
facs.save()
We don’t find one for “CyToF”, however, so, let’s create it without importing from a public ontology but label it as a child of “is_cytometry_assay”:
cytof = lb.ExperimentalFactor(name="CyTOF")
cytof.save()
is_cytometry_assay = lb.ExperimentalFactor(name="is_cytometry_assay")
is_cytometry_assay.save()
cytof.parents.add(is_cytometry_assay)
facs.parents.add(is_cytometry_assay)
is_cytometry_assay.view_parents(with_children=True)
Let us look at the content of the registry:
lb.ExperimentalFactor.filter().df()
name | ontology_id | abbr | synonyms | description | molecule | instrument | measurement | bionty_source_id | updated_at | created_by_id | |
---|---|---|---|---|---|---|---|---|---|---|---|
id | |||||||||||
lh5Cxy8w | fluorescence-activated cell sorting | EFO:0009108 | None | FACS|FAC sorting | A Flow Cytometry Assay That Provides A Method ... | None | None | None | WU6P | 2023-10-10 15:45:27 | DzTjkKse |
p8IsXqnb | CyTOF | None | None | None | None | None | None | None | None | 2023-10-10 15:45:27 | DzTjkKse |
5V3WHqSX | is_cytometry_assay | None | None | None | None | None | None | None | None | 2023-10-10 15:45:27 | DzTjkKse |
Register: data & annotate with metadata
#
modalities = ln.Modality.lookup()
features = ln.Feature.lookup()
experimental_factors = lb.ExperimentalFactor.lookup()
species = lb.Species.lookup()
file = ln.File.from_anndata(
adata, description="Alpert19", field=lb.CellMarker.name, modality=modalities.protein
)
... storing '$PnE' as categorical
... storing '$PnR' as categorical
file.save()
Inspect the registered file#
Inspect features on a high level:
file.features
Features:
var: FeatureSet(id='JHcIceelAnmy3piMNaZI', n=35, type='number', registry='bionty.CellMarker', hash='ldY9_GmptHLCcT7Nrpgo', updated_at=2023-10-10 15:45:28, modality_id='3TDhLk86', created_by_id='DzTjkKse')
'CD20', 'Cd14', 'CD27', 'CD94', 'CD38', 'Cd19', 'CD161', 'Ccr6', 'CD33', 'ICOS', 'CD24', 'TCRgd', 'CD25', 'Cd4', 'CXCR5', 'CD57', 'PD1', 'CD11B', 'CD85j', 'CD86', ...
obs: FeatureSet(id='P9uMy6qwTGgqSC57SXSB', n=5, registry='core.Feature', hash='HZvsq45MXE5c1h84OUmQ', updated_at=2023-10-10 15:45:28, modality_id='UxkvT8BD', created_by_id='DzTjkKse')
Cell_length (number)
Dead (number)
(Ba138)Dd (number)
Bead (number)
Time (number)
Inspect low-level features in .var
:
file.features["var"].df().head()
name | synonyms | gene_symbol | ncbi_gene_id | uniprotkb_id | species_id | bionty_source_id | updated_at | created_by_id | |
---|---|---|---|---|---|---|---|---|---|
id | |||||||||
cFJEI6e6wml3 | CD20 | MS4A1 | 931 | A0A024R507 | uHJU | Fbnq | 2023-10-10 15:45:22 | DzTjkKse | |
roEbL8zuLC5k | Cd14 | CD14 | 4695 | O43678 | uHJU | Fbnq | 2023-10-10 15:45:22 | DzTjkKse | |
uThe3c0V3d4i | CD27 | CD27 | 939 | P26842 | uHJU | Fbnq | 2023-10-10 15:45:22 | DzTjkKse | |
0qCmUijBeByY | CD94 | KLRD1 | 3824 | Q13241 | uHJU | Fbnq | 2023-10-10 15:45:22 | DzTjkKse | |
CR7DAHxybgyi | CD38 | CD38 | 952 | B4E006 | uHJU | Fbnq | 2023-10-10 15:45:22 | DzTjkKse |
Use auto-complete for marker names in the var
featureset:
markers = file.features["var"].lookup()
markers.cd14
CellMarker(id='roEbL8zuLC5k', name='Cd14', synonyms='', gene_symbol='CD14', ncbi_gene_id='4695', uniprotkb_id='O43678', updated_at=2023-10-10 15:45:22, species_id='uHJU', bionty_source_id='Fbnq', created_by_id='DzTjkKse')
In a plot, we can now easily also show gene symbol and Uniprot ID:
import scanpy as sc
sc.pp.pca(adata)
sc.pl.pca(
adata,
color=markers.cd14.name,
title=(
f"{markers.cd14.name} / {markers.cd14.gene_symbol} /"
f" {markers.cd14.uniprotkb_id}"
),
)
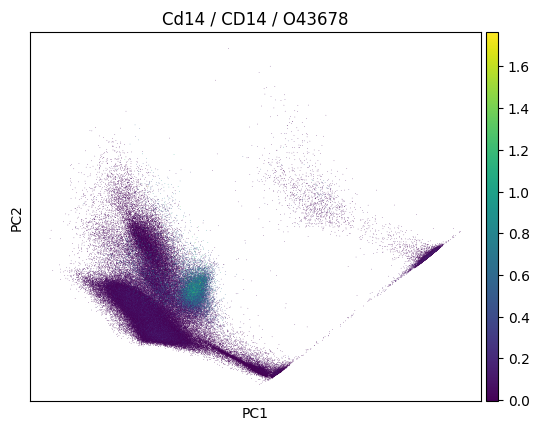
file.view_flow()
Create a dataset from the file#
dataset = ln.Dataset(file, name="My versioned cytometry dataset", version="1")
dataset
Dataset(id='QerZGf4taLaLa0UCUjEO', name='My versioned cytometry dataset', version='1', hash='VsTnnzHN63ovNESaJtlRUQ', transform_id='OWuTtS4SAponz8', run_id='R7aH6AfJFQF8f46JiC2H', file_id='QerZGf4taLaLa0UCUjEO', created_by_id='DzTjkKse')
Let’s inspect the features measured in this dataset which were inherited from the file:
dataset.features
Features:
var: FeatureSet(id='JHcIceelAnmy3piMNaZI', n=35, type='number', registry='bionty.CellMarker', hash='ldY9_GmptHLCcT7Nrpgo', updated_at=2023-10-10 15:45:28, modality_id='3TDhLk86', created_by_id='DzTjkKse')
'CD20', 'Cd14', 'CD27', 'CD94', 'CD38', 'Cd19', 'CD161', 'Ccr6', 'CD33', 'ICOS', 'CD24', 'TCRgd', 'CD25', 'Cd4', 'CXCR5', 'CD57', 'PD1', 'CD11B', 'CD85j', 'CD86', ...
obs: FeatureSet(id='P9uMy6qwTGgqSC57SXSB', n=5, registry='core.Feature', hash='HZvsq45MXE5c1h84OUmQ', updated_at=2023-10-10 15:45:28, modality_id='UxkvT8BD', created_by_id='DzTjkKse')
Cell_length (number)
Dead (number)
(Ba138)Dd (number)
Bead (number)
Time (number)
This looks all good, hence, let’s save it:
dataset.save()
Annotate by linking cytof & species labels:
dataset.labels.add(experimental_factors.cytof, features.assay)
dataset.labels.add(species.human, features.species)
dataset.view_flow()